VIDEO - HPC+AI@Edge Enabled X-ray Science at The Advanced Photon Source, with Mathew J. Cherukara
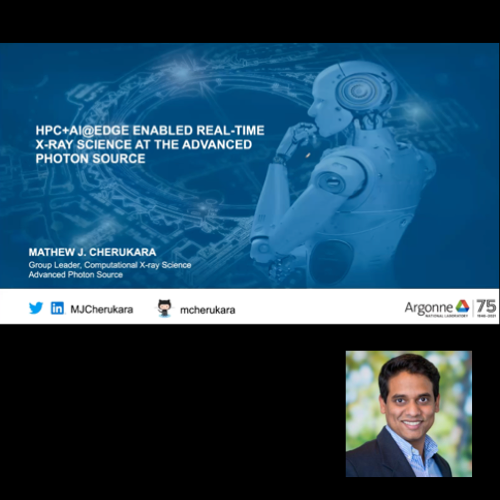

VIDEO - HPC+AI@Edge Enabled X-ray Science at The Advanced Photon Source, with Mathew J. Cherukara
CoWork webinar series
Speaker: Mathew Cherukara
The CoWork webinar series is dedicated to the exploitation of the coherence properties of X-rays for advanced materials characterization, with a special focus on inverse microscopy techniques, such as Coherent Diffraction Imaging (CDI), Ptychography and Holography. It is an introduction to Coherent X-ray imaging methods to facilitate the access to advanced microscopy techniques to new users and it welcomes all researchers intrigued by the spectacular coherence properties of X-rays produced at modern synchrotron sources – of which MAX IV is a first example.
Bio:
Mathew Cherukara leads the Computational X-ray Science (CXS) group at the Advanced Photon Source (APS) at Argonne National Laboratory. The group develops algorithms, computational tools and AI/ML models to analyze and interpret data from the various x-ray characterization techniques performed at the APS. His personal research is in AI-enabled materials characterization, AI-guided autonomous experiments and AI-accelerated materials modeling. He has particular interest in the development of novel x-ray and electron imaging capabilities that are only made possible because of AI. Mathew received his Ph.D from Purdue University in 2015 in computational materials science and his bachelors from the Indian Institute of Technology (IIT) Madras in 2010. He has 4 patents and has published over 50 peer-reviewed papers.
Abstract:
The capabilities provided by next generation light sources such as the APSU along with the development of new characterization techniques and detector advances are expected to revolutionize materials characterization (metrology) by providing the ability to perform scale-bridging, multi-modal materials characterization under in-situ and operando conditions. For example, providing the ability to image in 3D large fields of view (~mm3) at high resolution (~10 nm), while simultaneously acquiring information about structure, strain, elemental composition, oxidation state, photovoltaic response etc. However, these novel capabilities dramatically increase the complexity and volume of data generated by instruments at the new light sources. Conventional data processing and analysis methodologies become infeasible in the face of such large and varied data streams. The use of AI/ML methods is becoming indispensable for real-time analysis, data abstraction and decision making at advanced synchrotron light sources such as the APS. I will describe the use of high-performance computing (HPC) along with AI on edge devices to enable real-time analysis and autonomous experimentation on x-ray imaging instruments at the APS. References: 1. Babu, Anakha V., et al. "Deep learning at the edge enables real-time streaming ptychographic imaging." arXiv preprint arXiv:2209.09408 (2022). 2. Horwath, James P., et al. "Elucidation of Relaxation Dynamics Beyond Equilibrium Through AI-informed X-ray Photon Correlation Spectroscopy." arXiv preprint arXiv:2212.03984 (2022). 3. Yao, Yudong, et al. "AutoPhaseNN: unsupervised physics-aware deep learning of 3D nanoscale Bragg coherent diffraction imaging." npj Computational Materials 8.1 (2022): 1-8. 4. Chan, Henry, et al. "Rapid 3D nanoscale coherent imaging via physics-aware deep learning." Applied Physics Reviews 8.2 (2021): 021407. 5. Cherukara, Mathew J., et al. "AI-enabled high-resolution scanning coherent diffraction imaging." Applied Physics Letters 117.4 (2020): 044103. 6. Cherukara, M. J., Nashed, Y. S., & Harder, R. J. (2018). Real-time coherent diffraction inversion using deep generative networks. Scientific reports, 8(1), 1-8.